Large action models explained: Myths, realities, and their impact on AI evolution
October 18, 2024
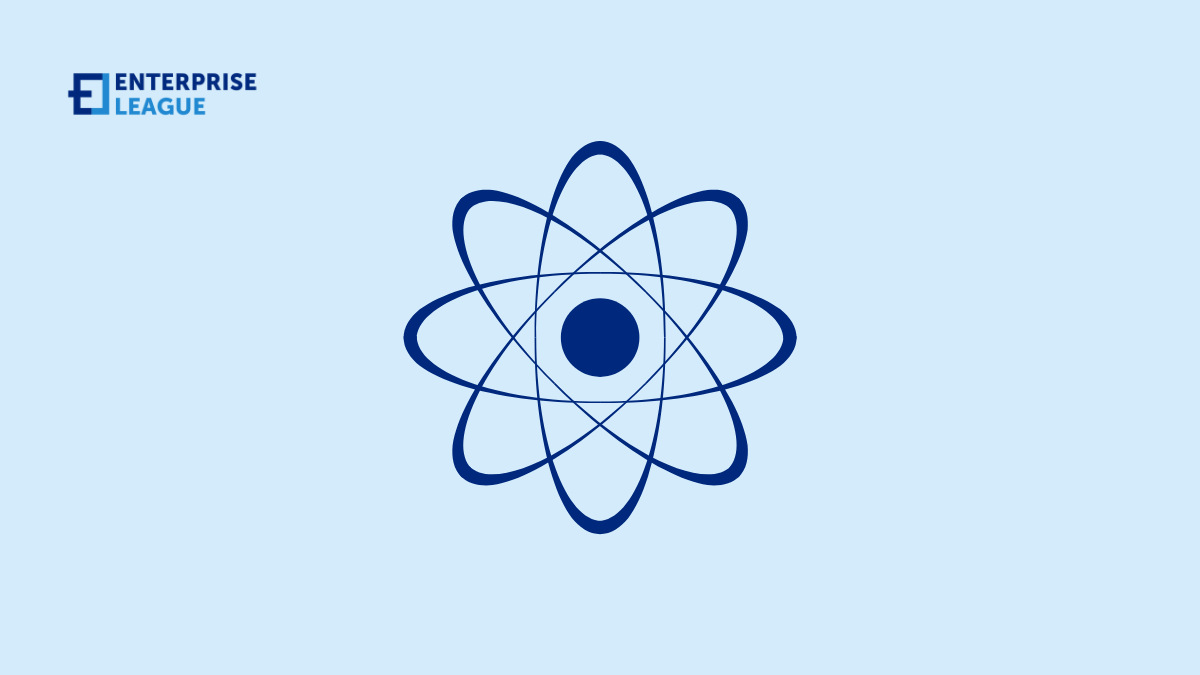
The rise of Large Action Models (LAMs) has sparked intense debate as artificial intelligence advances across industries. These sophisticated AI systems aim to transform our interactions with machines by executing diverse tasks based on their grasp of intricate environments. While LAMs offer exciting possibilities, they’ve also become a magnet for skepticism and misconceptions.
This article will explore the essence of LAMs, address common myths, examine the practical challenges of their implementation, and consider their far-reaching impact on AI’s future. Today, resilience is crucial for data management, making understanding these models even more vital for businesses.
What are large action models
Large Action Models refer to sophisticated AI systems designed to execute multiple tasks and make decisions based on large datasets and complex algorithms. Unlike traditional models that focus on narrow tasks, like classifying images or generating text, LAMs are built to adapt and respond dynamically to their environments. They leverage vast amounts of data, including insights from Large Language Models (LLMs), to understand patterns, predict outcomes, and take actions that align with their objectives.
LAMs are AI systems that go beyond just observing or analyzing – they take action. Imagine an AI that can run tasks, make choices, and streamline work in ways we’ve never seen before. These smart-systems use advanced learning methods and powerful computers to get better with practice. This makes them incredibly useful in many fields, from moving goods around to helping in hospitals. They’re like super-smart assistants who can handle complex jobs and keep improving as they work.
Myths surrounding large action models
Despite their potential, misconceptions about Large Action Models abound. Here are some prevalent myths:
- LAMs work on their own: Many think LAMs can make decisions without human input. The truth is, while they can automate tasks, they still need people to guide, train, and check their work.
- LAMs will take over human jobs: This isn’t the case. LAMs are built to enhance what humans can do, not replace them. They handle repetitive or data-heavy tasks, freeing up people to tackle more complex challenges.
- All LAMs are the same: LAMs actually vary widely. Some are designed for specific industries or jobs, while others are more general. It’s crucial to understand these differences when using LAMs.
- LAMs are always right: While LAMs can be very accurate, they’re not perfect. Their results depend on the quality of data they’re trained with. If that data is biased or incomplete, LAMs can make mistakes.
Realities of large action models
Now that we’ve dispelled some common misconceptions and myths, let’s examine the current state of LAM technology, its real-world applications, and the challenges that developers and users face when implementing these systems in various industries.
Data-driven insights and decision-making
LAMs thrive on data. They analyze vast datasets to derive insights and inform decision-making processes. This capability allows businesses to make informed choices based on trends, patterns, and predictive analytics. The reliance on data reinforces the need for resilience in data management, ensuring that the data feeding these models is accurate, comprehensive, and well-maintained.
Complex task execution
LAMs excel at executing complex tasks that involve multiple steps and decisions. For example, in supply chain management, a LAM can analyze inventory levels, predict demand, optimize routes, and automate ordering processes, all while adapting to real-time changes. This multifaceted capability makes them powerful tools for improving operational efficiency.
Integration with existing systems
One of the critical realities of implementing LAMs is their ability to integrate with existing systems and workflows. Organizations do not need to overhaul their entire infrastructure to adopt LAMs. Instead, these models can complement current technologies, enhancing performance without disruption.
Scalability
Large Action Models are built to grow alongside businesses. As companies expand, their data needs change, and LAMs can adjust to meet these new demands. This flexibility is especially valuable in fast-moving industries. It allows organizations to stay nimble and quickly adapt to shifts in the market. With LAMs, businesses can scale their AI capabilities without overhauling their entire system, making it easier to keep pace with growth and changing conditions.
The impact of LAMs on AI evolution
As we move further into the era of AI, Large Action Models are poised to have a significant impact on its evolution. Here are some of the critical implications:
Enhanced human-AI collaboration
With the ability to perform complex tasks and learn from interactions, LAMs can enhance collaboration between humans and AI. By automating routine tasks, these models free up human workers to focus on creative and strategic initiatives. This synergy fosters a more productive work environment where both parties contribute their strengths.
Driving innovation
The flexibility and adaptability of LAMs can drive innovation across industries. By enabling organizations to experiment with new approaches, products, and services, LAMs encourage a culture of creativity and exploration. Companies can test ideas in real time, gather feedback, and iterate on their offerings more efficiently.
Improved operational efficiency
Large language models (LLMs) are revolutionizing business operations. By leveraging these AI tools, companies can analyze vast datasets, forecast trends, and automate complex tasks. This leads to streamlined processes, reduced expenses, and fewer human errors. As a result, businesses employing LLMs are likely to see marked improvements in productivity and competitiveness.
Resilience in data management
Today, resilience is crucial for data management. Large language models (LLMs) enhance this adaptability by enabling organizations to swiftly process and respond to changes in their data environment. These AI systems can rapidly analyze new information from various sources, such as shifting market trends or supply chain disruptions.
By providing timely insights and recommendations, LLMs help businesses proactively adjust their strategies, manage risks, and maintain a competitive edge in dynamic markets.
Use cases of large action models in practice
To further illustrate the impact of large language models (LLMs), it’s helpful to examine some real-world applications. These examples demonstrate how LLMs are transforming various industries and business functions. The following instances highlight how organizations are leveraging LLMs to solve complex problems, enhance decision-making, and create innovative solutions across different sectors:
- Healthcare management: LAMs can analyze patient data to predict outcomes, streamline patient care, and optimize resource allocation in hospitals.
- Supply chain optimization: By analyzing logistics data, LAMs can predict delays, manage inventory levels, and optimize delivery routes.
- Fraud detection: In finance, LAMs can monitor transaction patterns to identify anomalies that may indicate fraudulent activity.
- Customer service automation: LAMs can power chatbots and virtual assistants, providing instant responses to customer inquiries while learning from interactions to improve over time.
Conclusion
Large Action Models (LAMs) are poised to revolutionize artificial intelligence, offering powerful capabilities that can significantly enhance business efficiency and decision-making. Despite some misconceptions, their potential to improve operational performance and foster innovation is clear. As organizations continue to embrace AI technologies, understanding and leveraging LAMs will be crucial for adapting to a rapidly evolving digital landscape and achieving long-term success.
More must-read stories from Enterprise League:
- Unique ways to show your employees you care about them.
- Why hiring millennials might be the best decision you can ever make.
- Things to consider before deciding on a business location.
- Getting your product in stores doesn’t have to be complicated.
- Innovative small business growth tips that will take you to the next level.
Related Articles
How Do I Choose the Best Software for Municipal Waste Management?
Integrating waste management software marks a crucial evolution that can dramatically enhance your operational performance. Research shows that municipalities implementing smart waste solutions have experienced operational cost savings of up to 30% while boosting...
Top-Rated Safety Training Programs for Forklift Operators
Forklifts are crucial to various industries, such as construction, warehouses, logistics, and manufacturing. There are multiple types, and operating them requires expertise to ensure workplace safety and efficiency. According to the Occupational Safety and Health...
What banks are getting completely wrong about FX transactions?
Forex transactions have become ubiquitous in the modern globalized financial system, with over $7.5 trillion traded daily in the forex market as of 2024. Millions of individuals and institutions enter the market to exchange one currency for another. However, depending...
How Do I Choose the Best Software for Municipal Waste Management?
Integrating waste management software marks a crucial evolution that can dramatically enhance your operational performance. Research shows that municipalities implementing smart waste solutions have experienced operational cost savings of up to 30% while boosting...
Top-Rated Safety Training Programs for Forklift Operators
Forklifts are crucial to various industries, such as construction, warehouses, logistics, and manufacturing. There are multiple types, and operating them requires expertise to ensure workplace safety and efficiency. According to the Occupational Safety and Health...