Scaling your data annotation efforts: A guide to efficient labeling
April 23, 2024
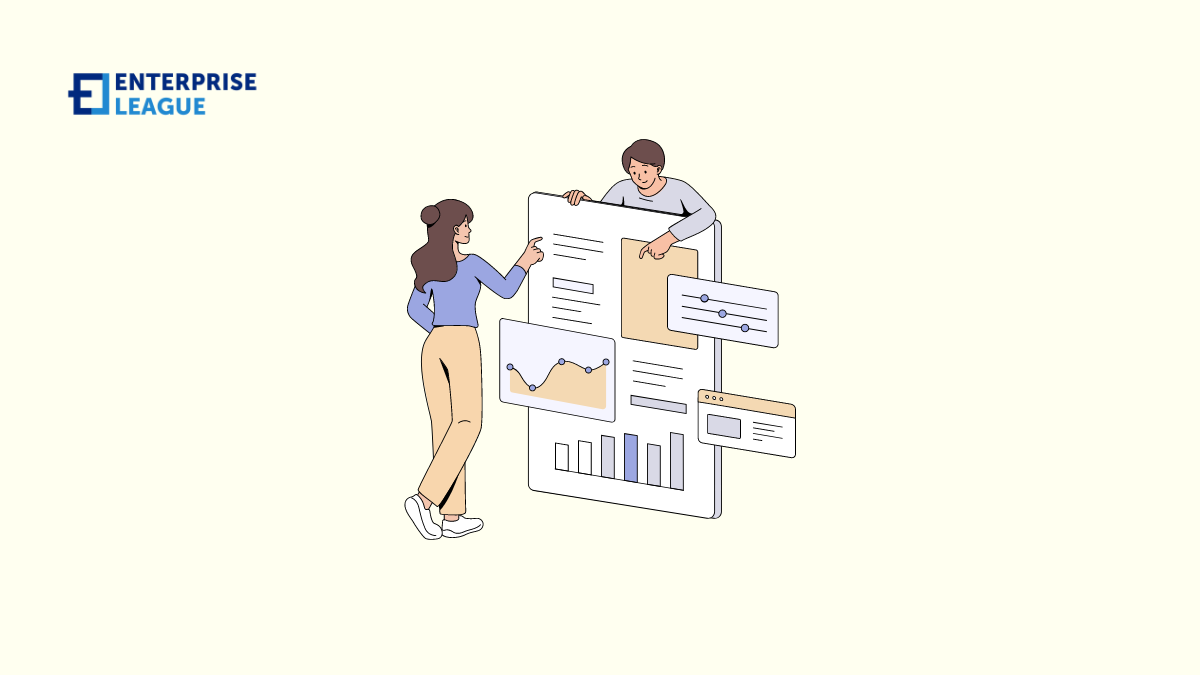
While working with AI projects, the capability to scale data annotation efficiently stands as a cornerstone for success. This guide unpacks essential strategies for expanding annotation efforts without sacrificing quality. It highlights the critical role of a thought-out data labeling strategy, digs into workflow optimization, and presents actionable steps for scaling up.
The foundation of data annotation
By 2030, AI has the potential to generate an extra $13 trillion in global economic activity, according to McKinsey. Such growth hinges on high-quality data annotation, the backbone of AI’s learning process. The foundation of any successful data annotation project lies in a robust data labeling strategy. This strategy ensures AI models train on accurate and relevant data. Moreover, it streamlines the annotation process, significantly impacting efficiency and outcome.
Defining clear objectives at the outset forms the cornerstone of an effective data labeling strategy. Objectives guide the choice of tools and methods, ensuring they align with the project’s needs. Furthermore, understanding your project’s specific requirements allows for a tailored approach. This not only optimizes the process but also conserves valuable resources like time and money.
Here are key elements to consider in your strategy:
- Goal alignment: Ensure the project’s objectives match the intended outcomes of the AI model.
- Data quality: Focus on collecting high-quality data that is representative of real-world scenarios.
- Tool selection: Choose annotation tools that offer the right balance of efficiency, accuracy, and scalability.
- Team expertise: Leverage skilled annotators who can understand and execute your project’s unique requirements.
Well-planned data labeling strategy serves as the blueprint for successful data annotation projects. It not only ensures that your AI models train on high-quality data but also streamlines the entire process. This strategic approach ultimately saves time and resources.
How to optimize data labeling operations
Optimizing data labeling operations requires a multifaceted approach, especially as you scale. Efficient scaling means increasing your annotation output without losing quality. Let’s go through the best practices that ensure this balance.
First, selecting the right tools stands paramount. Choosing the right one should align with your project’s specific needs. Look for tools that provide:
- Customization options for different data types.
- Integration capabilities with your existing workflow.
- Scalability to handle growing data volumes.
Next, maintaining high-quality datasets throughout the annotation process is vital. This starts with rigorous data cleaning and preprocessing. Ensure the data is free from errors or irrelevant information before it even reaches the annotation stage. This not only improves the quality of the training data but also the efficiency of the annotators.
Implementing effective quality control measures further safeguards the integrity of your annotated data. Regular audits and reviews by experienced annotators can catch and correct errors early. Additionally, incorporating a tiered review system, where multiple annotators review each piece of data, enhances accuracy.
Automated tools and AI-assisted annotation techniques present significant advantages in scaling efforts. These technologies can:
- Accelerate the annotation process by pre-labeling data.
- Reduce the workload on human annotators, allowing them to focus on more complex tasks.
- Improve consistency across large datasets.
However, the importance of a skilled workforce cannot be overstated. Even the most advanced AI-assisted tools require human oversight. Investing in training for your annotation team ensures they are up-to-date with the latest tools and techniques. It also fosters a deeper understanding of the nuances in the data, leading to higher-quality annotations.
Optimizing data labeling operations as you scale involves a combination of the right tools, stringent quality controls, leveraging technology, and investing in your team. This holistic approach ensures your data annotation efforts are not just about expanding in volume but also enhancing in value.
Practical steps to scale your data annotation efforts
As your project grows from a small-scale operation to handling vast amounts of data, following these practical steps can ensure success in your data labeling operations at scale.
- Start with a pilot: Before full-scale implementation, run a pilot project. This allows you to identify potential bottlenecks and adjust your strategies accordingly.
- Select scalable tools: Choose annotation tools that can easily scale up. Look for features such as cloud-based platforms, automation capabilities, and easy integration with other software.
- Build a skilled team: As the volume of data grows, so does the need for a larger annotator team. Focus on hiring skilled personnel. Provide ongoing training to keep them abreast of new technologies and methodologies.
- Implement quality control: Maintain high-quality standards by establishing a robust quality control system. This should include multiple levels of checks and balances to ensure accuracy.
- Monitor and adjust: Use metrics to monitor progress and performance. Key performance indicators (KPIs) might include annotation speed, accuracy rates, and project completion times. Analyze these metrics regularly to identify areas for improvement.
- Automate where possible: Incorporate AI-assisted tools to pre-label data, reducing the manual workload and increasing consistency across large datasets.
- Foster communication: Ensure clear and open communication channels within your team. This helps in quickly resolving issues and sharing best practices.
These strategies enable you to handle larger datasets while maintaining the quality of your annotations, crucial for the success of AI applications
Conclusion
Scaling your data annotation efforts effectively hinges on strategic planning, leveraging the right tools, and building a skilled team. From initiating a pilot to monitoring and adjusting your strategies, each step is crucial for enhancing quality.
Adopting these practices ensures that your data labeling operations at scale meet the growing demands of AI applications without compromise. Remember, continuous improvement through regular monitoring and adaptation to feedback plays a pivotal role in success.
More must-read stories from Enterprise League:
- Learn about all the tips and apps you need to successfully manage a remote team.
- Motivating business role models to inspire your entrepreneurial spirit.
- Engaging online networking events that you should not miss.
- Engaging online networking events that you should not miss.
Related Articles
How to achieve consistent product quality on a production line with digital solutions
From automated inspections to data-driven decisions, lets see how you can achieve consistent product quality on a production line with digital solutions.
Top 16 email security startups you should know in 2025
From inbox monitoring to automated threat response, these innovative email security startups are building the future of digital communication protection.
Hybrid, remote or in-person: Which work model is right for you?
In this article, you will learn about the unique features of each work model and the key factors to consider when deciding which one suits your needs.
How to achieve consistent product quality on a production line with digital solutions
From automated inspections to data-driven decisions, lets see how you can achieve consistent product quality on a production line with digital solutions.
Top 16 email security startups you should know in 2025
From inbox monitoring to automated threat response, these innovative email security startups are building the future of digital communication protection.